People analytics or insights. Talent analytics or insights. Workforce analytics or insights.
However you define the field of pairing data with human resources, the end-result remains the same: Making HR more strategic, more impactful to the business as a whole. How can an HR professional use data modeling to predict and demonstrate ROI on a recruitment process, retention capability, productivity levels, adherence to compliance, overall work culture? By incorporating data into each HR decision, professionals are better able to get a seat at the C-suite, making solid recommendations to senior management and key decision makers because they have data to back up their proposals.
According to a recent report from Deloitte, more companies are investing heavily in people analytics teams and the technology to support these professionals. "Fully 77 percent of all organizations believe people analytics is important," the report states. "And more than half of the organizations now rate themselves as excellent and 38 percent as adequate at conducting multi-year workforce planning."
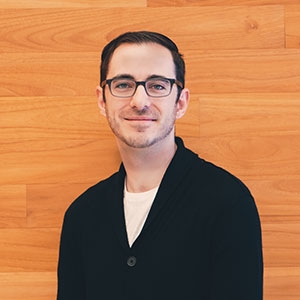
With data becoming an increasingly important aspect of HR strategy, we recently sat down with Certificate Program in Human Resource Management required course Introduction to Human Capital Analytics instructor's, Will Gaker, M.A., who is senior manager in business operations and analytics at LinkedIn for the past three years. He uses human capital analytics to help organizations build a business case for employees, who then are empowered to create even greater business value. Prior to LinkedIn, he worked at several Fortune 100 companies where he created analytics training programs and applied analytics across the employee lifecycle.
How did you get into this field?
I stumbled into it by accident. When I was in undergrad, figuring out what I wanted to do with my career, I knew I did not want to become a numbers guy. I wanted to be a people person: someone who helps empower managers and workers and connects them to opportunities. So I studied psychology as a way to learn more about people and social science research methods. I realized that numbers are really powerful because it's a whole new way to tell a story. You can look at people in groups and look between the lines of what the numbers are telling you.
That led me to pursue a graduate degree in industrial psychology, which also brought me out to California. I was so passionate about statistics and analytics that I ended up taking more research classes than psychology classes. I started looking around for internships and I ended up finding a job in a department doing what they called HR Analytics at the time. I've been doing it ever since and I've enjoyed every minute of it.
Data helps HR speak the language of the business. It gives HR a seat at the table.
Why is people analytics such an important tool for HR professional today?
It empowers HR to do a number of things differently. One, it helps HR speak the language of the business. When you think about it, 80 percent of an organization's cost is its workforce—it's an organization's greatest asset. With great tools like Success Factor and SAP and databases that allow us to structure and store information about our workers, we can be just as strategic and just as analytical as any other department of the business. It gives HR a seat at the table.
How does this ultimately optimize workplace efficiency and improve ROI?
First, it reduces the risk that we make a potentially bad decision at the leadership level because we're giving more information to our leaders. Using the data we provide, leadership is confident that the decisions they're making are the correct ones.
Analytics also gives you visibility into the areas of the business that you had no idea could be optimized. When you figure out a way to optimize those areas of the business, you end up with a practical ROI.
I'll give you an example that we had at LinkedIn: We were in a very high growth phase where recruiting was one of our top strategic priorities for our talent organization. We needed to supply the business with the employees who were going to scale LinkedIn and take our member base into tremendous growth. We were constantly revising our headcount numbers. That ended up being very challenging for our recruiting team because they were constantly playing catch-up: We had to hire more recruiters and onboard them, which made it hard for us to hit that head count goal. When we don't hit the head count goal, we don't have the workers who are going to help us solve our business challenges. So we started using analytics to forecast how much attrition we'd expect, how many hires we'd expect, on average how many recruiters it takes to fill a certain number of roles across the business.
That gave us a head-start on our headcount goals so we didn't have to wait until the end of Q1 or beginning of Q2 to hit that goal. We would start hiring recruiters in January, so that by the time we got our head count number the recruiters could hit the ground running and interview candidates. That allowed us to be really strategic about what recruiting talent we needed, when we needed it. And to make sure we had the right resources in the right places at the right time. Once we did that, we were able to come in under budget for our recruiting organization. That gave us such an ROI that it paid for our team for several years just through one project.
Behind every data point on a spreadsheet is a story, something happening in the business.
During that project, you're looking at a lot of raw data to come to business conclusions. What advice would you give to other professionals in how they can avoid pitfalls when aggregating data?
One of the biggest pitfalls you can run into when doing an analysis is not understanding the context that surrounds the data. Behind every data point on a spreadsheet is a story, something happening in the business. If you don't make an effort to really understand the environment and the landscape of the business, your insights could potentially become misleading.
I was doing an analysis of span of control, which is on average how many direct reports do our managers have. If that number is too small, then we have too vertical of an organization. If that number is too big, we have managers who are overloaded and their teams are not getting the coaching and support that they need to develop. I came across someone who had 52 direct reports in that spreadsheet. Had I trusted the data and not understood the context of the business, I would have gone straight to the leaders, and said first priority is making sure that we do not allow people to have 52 direct reports. And I would have gotten laughed at because it turns out that the person who had 52 direct reports was a program manager who managed our business leadership program. We were bringing in cohorts of new graduates and rotating them around the business to give them experience. After that program concluded, they would get a permanent manager. So this program manager's direct reports weren't true direct reports.
Asking myself the question, "What does this data mean? Based on what I know of the business, does this data make sense?" was a great way to check myself before going to a leader with misleading information.
When you're doing analytics, your credibility is your capital. People rely on you because they trust your analysis, your ability to connect the analysis to the business and to give them advice.
How do you measure employee value?
The Mecca of what we ultimately want to get to is being able to as precisely as we can measure the unique contributions and value that each employee provides to the organization. The challenge is that one value is subjective—it means different things to different businesses—so you have to understand what your culture, what your organization values in the workforce.
The other hard part is that you can measure value in a monetary way. For example, it's easy to measure the value the sales team produces because they're bringing in revenue. Or recruiting because you've got this quantitative outcome, as well as some operations roles because you can measure productivity or output. In other roles, it's a bit more challenging because the work that's being done is very qualitative in nature.
When you're studying value, you have to acknowledge that value means different things to different organizations, to different leaders, to different functions, to different departments. So when someone is asking you to measure value for them, you have to unlock their brain and ask them, "What does value mean to you?" Do we have the ability to measure whether that value is being created. And if we don't, could we implement something that will allow us to measure value so that our data ecosystem is becoming more mature and more robust over time. In my search for the silver bullet that measures value for everyone, what I found is that it's just different for organizations. You have to have set expectations with the people you're working with and have a shared definition of what value means so you can measure it.
Questions drive analysis. But analysis can create further questions.
Does that inform the types of questions you ask of the data?
Questions drive analysis, not the other way around. But analysis can create further questions. The amount of data that we have access to in this era of big data is overwhelming. It's like trying to find a needle in the haystack. But imagine trying to find a needle in the haystack when you didn't know you were looking for a needle. So the question really drives the focus in what you're trying to answer. It's very difficult to dive into a data set without having a question you're trying to answer because you don't know what to look for.
So the first problem is you need to have a question before you can run analysis. The second problem you run into is once you've started asking questions, there are so many questions and you can't get to all of them. Some of those questions will produce more value for the organization than others. Work with your leaders to identify questions; once they get started, they will throw as many questions at you as they can—it's great! Then work with them to prioritize those questions: What are the one or two questions that are going to fundamentally improve the way that your business is operating? Then you know exactly what to look for instead of looking for a needle in a haystack. You're looking for something much easier to find, like an anvil in a haystack.
Can anyone learn and succeed in this field without having a background in data science or statistics?
Let's assume you don't have a data science background. The field is about marrying analysis with context, and when you have those two ingredients that's the recipe for impact. If you don't have the background in data science, analytics or statistics, you could have a very rich knowledge of the business or the HR field. So even if you're not the one doing a complex regression analysis, estimating probability, or doing complex analytics, you can be the person who is a change advocate or the person who consumes that information and knows exactly what to do with it because you know the organization like the back of your hand.
This field is meant to empower HR.
This field is not meant to be a discouraging force within HR; it's meant to empower HR. There are people like myself who are very passionate about the statistics and the analytical side and enjoy applying it to the people-side of the business. But people like me need help; we need people who are mature, strategic HR professionals who know what to do with this information, how to ask us the right questions of the data, how to influence leaders and how to make change happen in an organization. Without those people, people like myself who are doing the analytics have an uphill battle to climb because once we get done with the analysis, phase 2—implementation—begins.
You bring a lot of your experience with people analytics to the Human Capital Analytics course. What excites you about teaching this fascinating topic?
I love the energy that students bring into a class and how eager and excited they are to begin this next phase of their life. They come in with a lot of optimism and enthusiasm. That's contagious and it fires me up when I'm at work.
When you come into the classroom on day 1, you have a lot of expectations around what you think the course is going to be: very math intensive or very dry and logical and not fun. But I love to make it fun by using real-world examples that people can easily understand. We don't do a ton of math in the class; we joke around, we try to make the activities fun and learn together.
What you learn the minute you start studying statistics or research is it's more logical thinking or systemic reasoning; computers do a majority of the work for us nowadays. I've had people come in with non-business backgrounds or who haven't studied statistics, and they've done very well in the course because it is focused on teaching you how to think strategically, how to break down a problem into digestible chunks so that you can solve it. We're not going to teach you complex advanced statistics that you would need tons of training for.
If you're really excited about the numbers, the logical reasoning and want to get involved in that aspect of the field, you've got a preview of that and what you need to learn to start that aspect of your career.